GitHub - likyoo/change_detection.pytorch: Deep learning models for change detection of remote sensing imageshttps://github.com/likyoo/change_detection.pytorch这个框架用起来很方便,下载以后基本不用改什么,直接就可以用,下面做个简要说明
1.下载数据,我下载了LEVIR-CD做测试
数据具体链接在这里LEVIR-CD | LEVIR-CD is a new large-scale remote sensing binary change detection dataset, which would help develop novel deep learning-based algorithms for remote sensing image change detection.LEVIR-CD is a new large-scale remote sensing binary change detection dataset, which would help develop novel deep learning-based algorithms for remote sensing image change detection.https://justchenhao.github.io/LEVIR/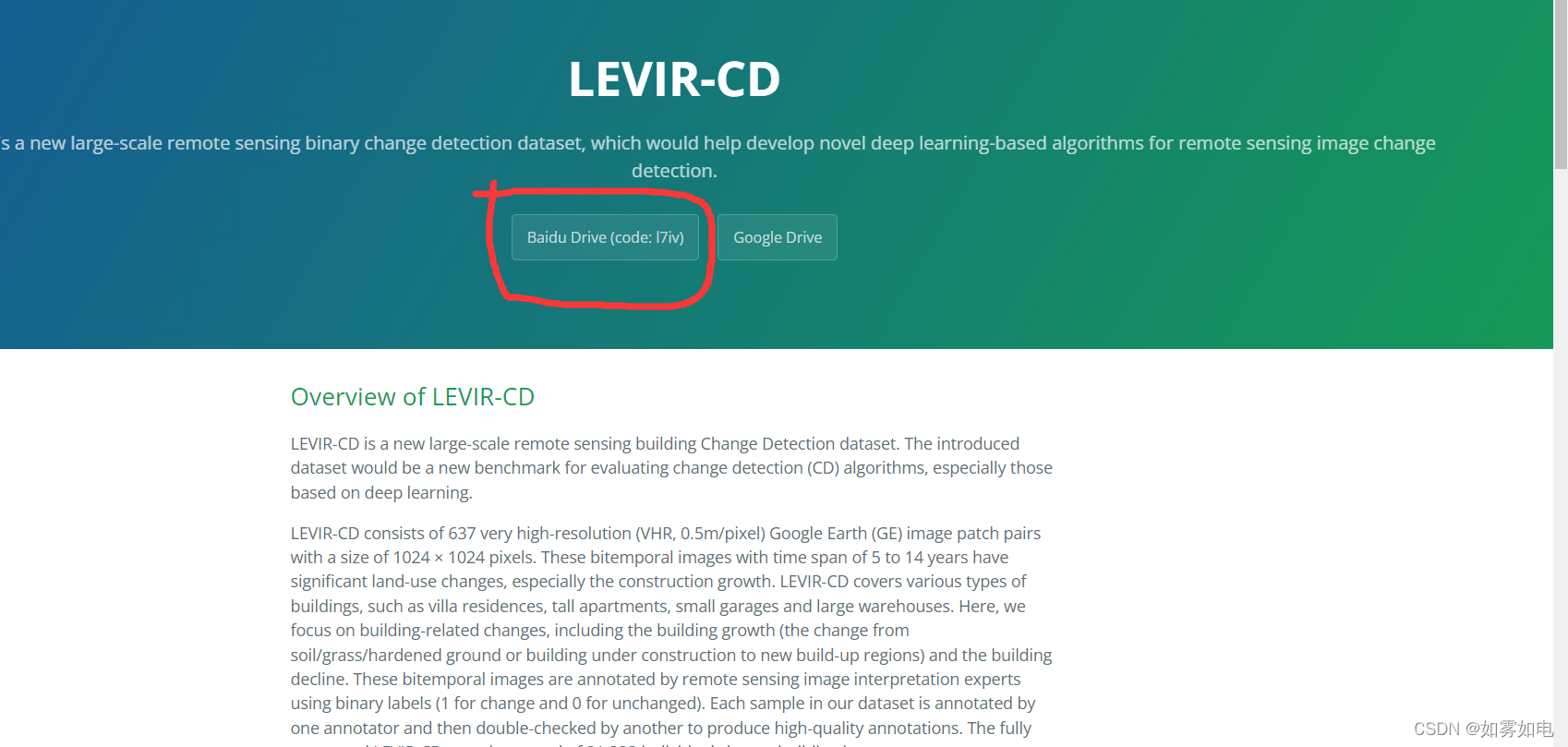
下载好了随便放哪里,放好了改下训练脚本的路径就可以了,解压了就可以用
2.训练,训练脚本(local_test.py)里把路径改了就可以直接运行了
训练完的权重默认就在根目录
3.预测,原作者没有直接给出预测代码,这里我贴一下我用的
import cv2
import numpy as np
import torch
from torch.utils.data import DataLoader, Dataset
import albumentations as A
import change_detection_pytorch as cdp
from change_detection_pytorch.datasets import LEVIR_CD_Dataset, SVCD_Dataset
from change_detection_pytorch.utils.lr_scheduler import GradualWarmupScheduler
DEVICE = 'cuda' if torch.cuda.is_available() else 'cpu'
model = cdp.Unet(
encoder_name="resnet34", # choose encoder, e.g. mobilenet_v2 or efficientnet-b7
encoder_weights="imagenet", # use `imagenet` pre-trained weights for encoder initialization
in_channels=3, # model input channels (1 for gray-scale images, 3 for RGB, etc.)
classes=2, # model output channels (number of classes in your datasets)
siam_encoder=True, # whether to use a siamese encoder
fusion_form='concat', # the form of fusing features from two branches. e.g. concat, sum, diff, or abs_diff.
)
model_path = './weights/best_model.pth'
model.to(DEVICE)
# model.load_state_dict(torch.load(model_path))
model = torch.load(model_path)
model.eval()
test_transform = A.Compose([
A.Normalize()])
path1 = './change_detection_pytorch/LEVIR_CD/test/A/test_7.png'
img1 = cv2.imread(path1)
img1 = test_transform(image = img1)
img1 = img1['image']
img1 = img1.transpose(2, 0, 1)
img1 = np.expand_dims(img1,0)
img1 = torch.Tensor(img1)
img1 = img1.cuda()
path2 = './change_detection_pytorch/LEVIR_CD/test/B/test_7.png'
img2 = cv2.imread(path2)
img2 = test_transform(image = img2)
img2 = img2['image']
img2 = img2.transpose(2, 0, 1)
img2 = np.expand_dims(img2,0)
img2 = torch.Tensor(img2)
img2 = img2.cuda()
pre = model(img1,img2)
pre = torch.argmax(pre, dim=1).cpu().data.numpy()
cv2.imwrite('./result/test_7_pre.png', pre[0])
结果
A B
标签 预测结果
版权归原作者 如雾如电 所有, 如有侵权,请联系我们删除。