1、简介
** ** Inception 网络是卷积神经网络发展史上一个重要的里程碑。在 Inception 网络出现之前,大部分流行卷积神经网络都是把卷积层堆叠得越来越多,使网络越来越深,来对特征进行多次处理,以此希望能够得到更好的性能。Inception网络对输入图像进行并行采集特征,并将所有输出结果拼接为一个非常深的特征图****,由于并行提取特征时卷积核大小不一样,这也就在一定程度上丰富了特征,使特征多样化。
2、inception网络结构
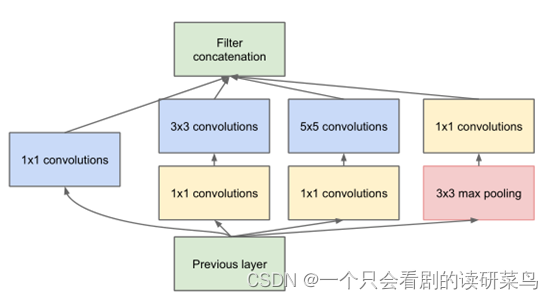
类似上述这种并行采集信号特征的方式,输入一张图片进来,会有1*1、3*3、5*5等不同大小的卷积核进行特征提取。这样,**网络中每一层都能学习到不同的特征**,既增加了网络的宽度,也增加了网络对尺度的适应性。使用1x1的卷积核实现降维操作,以此来减小网络的参数量。
网络结构CBAPD如下:
** 可以看出卷积都是使用全零填充,而激活函数都是 relu,基本都是CPA的结构,没有池化层和丢失层。**
3、python实现
采用的数据集还是fashion数据集,可以自己去深刻了解。
主要结构:
class ConvBNRelu(Model):
def __init__(self, ch, kernelsz=3, strides=1, padding='same'):
super(ConvBNRelu, self).__init__()
self.model = tf.keras.models.Sequential([
Conv2D(ch, kernelsz, strides=strides, padding=padding),
BatchNormalization(),
Activation('relu')
])
def call(self, x):
x = self.model(x, training=False)
return x
class InceptionBlk(Model):
def __init__(self, ch, strides=1):
super(InceptionBlk, self).__init__()
self.ch = ch
self.strides = strides
self.c1 = ConvBNRelu(ch, kernelsz=1, strides=strides)
self.c2_1 = ConvBNRelu(ch, kernelsz=1, strides=strides)
self.c2_2 = ConvBNRelu(ch, kernelsz=3, strides=1)
self.c3_1 = ConvBNRelu(ch, kernelsz=1, strides=strides)
self.c3_2 = ConvBNRelu(ch, kernelsz=5, strides=1)
self.p4_1 = MaxPool2D(3, strides=1, padding='same')
self.c4_2 = ConvBNRelu(ch, kernelsz=1, strides=strides)
def call(self, x):
x1 = self.c1(x)
x2_1 = self.c2_1(x)
x2_2 = self.c2_2(x2_1)
x3_1 = self.c3_1(x)
x3_2 = self.c3_2(x3_1)
x4_1 = self.p4_1(x)
x4_2 = self.c4_2(x4_1)
# concat along axis=channel
x = tf.concat([x1, x2_2, x3_2, x4_2], axis=3)
return x
class Inception(Model):
def __init__(self, num_blocks, num_classes, init_ch=16, **kwargs):
super(Inception, self).__init__(**kwargs)
self.in_channels = init_ch
self.out_channels = init_ch
self.num_blocks = num_blocks
self.init_ch = init_ch
self.c1 = ConvBNRelu(init_ch)
self.blocks = tf.keras.models.Sequential()
for block_id in range(num_blocks):
for layer_id in range(2):
if layer_id == 0:
block = InceptionBlk(self.out_channels, strides=2)
else:
block = InceptionBlk(self.out_channels, strides=1)
self.blocks.add(block)
# enlarger out_channels per block
self.out_channels *= 2
self.p1 = GlobalAveragePooling2D()
self.f1 = Dense(num_classes, activation='softmax')
def call(self, x):
x = self.c1(x)
x = self.blocks(x)
x = self.p1(x)
y = self.f1(x)
return y
model = Inception(num_blocks=2, num_classes=10)
添加库和读取数据集 :
import tensorflow as tf
import os
import numpy as np
from matplotlib import pyplot as plt
from tensorflow.keras.layers import Conv2D, BatchNormalization, Activation, MaxPool2D, Dropout, Flatten, Dense, \
GlobalAveragePooling2D
from tensorflow.keras import Model
np.set_printoptions(threshold=np.inf)
fashion = tf.keras.datasets.fashion_mnist
(x_train, y_train), (x_test, y_test) = fashion.load_data()
x_train, x_test = x_train / 255.0, x_test / 255.0
x_train = x_train.reshape(x_train.shape[0], 28, 28, 1)
x_test = x_test.reshape(x_test.shape[0], 28, 28, 1)
迭代求解并绘图:
model.compile(optimizer='adam',
loss=tf.keras.losses.SparseCategoricalCrossentropy(from_logits=False),
metrics=['sparse_categorical_accuracy'])
cp_callback = tf.keras.callbacks.ModelCheckpoint(filepath=checkpoint_save_path,
save_weights_only=True,
save_best_only=True)
history = model.fit(x_train, y_train, batch_size=128, epochs=20, validation_data=(x_test, y_test), validation_freq=1,
callbacks=[cp_callback])
model.summary()
acc = history.history['sparse_categorical_accuracy']
val_acc = history.history['val_sparse_categorical_accuracy']
loss = history.history['loss']
val_loss = history.history['val_loss']
plt.subplot(1, 2, 1)
plt.plot(acc, label='Training Accuracy')
plt.plot(val_acc, label='Validation Accuracy')
plt.title('Training and Validation Accuracy')
plt.legend()
plt.subplot(1, 2, 2)
plt.plot(loss, label='Training Loss')
plt.plot(val_loss, label='Validation Loss')
plt.title('Training and Validation Loss')
plt.legend()
plt.show()
结果如下图:
版权归原作者 似水不惧 所有, 如有侵权,请联系我们删除。